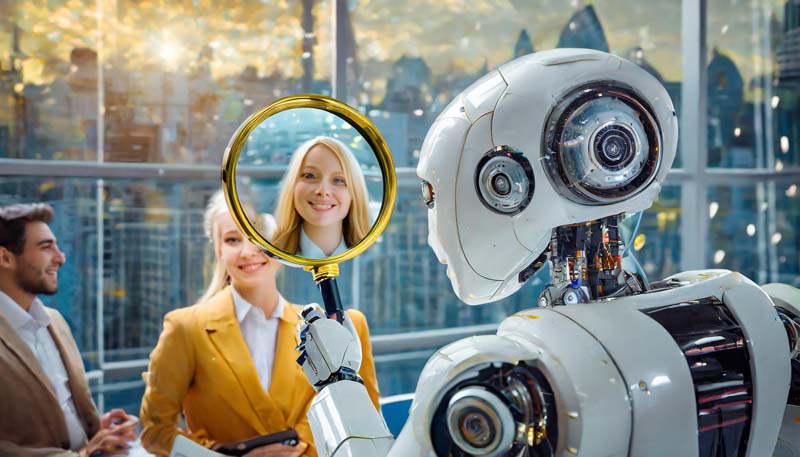
Using AI to Decode Freemium User Actions
Exploring the role of AI in understanding user behaviour in freemium services.
The Freemium Business Model Explained
Welcome to our in-depth exploration of the freemium business model! In this article, we will delve into the key aspects of freemium businesses, including their definition, successful examples, the psychology behind the model and user engagement, and the importance of user behavior analysis. Let’s jump right in!
Definition and Examples of Successful Freemium Businesses
Firstly, let’s clarify what exactly the freemium business model entails. Freemium is a pricing strategy where a basic version of a product or service is offered for free, while advanced features or premium content are available for a price. This approach aims to attract a large user base with the free offering and convert a percentage of them into paying customers for the premium features.
There are several successful examples of companies that have effectively implemented the freemium model. One prominent example is Dropbox, a cloud storage service that offers free storage space with the option to upgrade for additional features and storage capacity. Another well-known freemium success story is Spotify, a music streaming platform that provides free, ad-supported access to music and offers a premium subscription for an ad-free experience and additional perks.
Psychology Behind Freemium Model and User Engagement
The freemium model leverages various psychological principles to engage users and convert them into paying customers. By offering a free version of the product or service, businesses can tap into the concept of reciprocity, where users feel inclined to reciprocate the value they have received by upgrading to the premium offering. Additionally, the lure of exclusive features or content available only to premium subscribers can create a sense of scarcity and exclusivity, driving users to upgrade.
Furthermore, the freemium model capitalizes on the psychological phenomenon of loss aversion, where users are more motivated by the fear of losing access to a valuable service than by the potential gains of upgrading. By providing a taste of the benefits of the premium offering through the free version, businesses can stimulate users’ desire to avoid losing those benefits, encouraging them to upgrade.
Importance of User Behavior Analysis in Freemium Models
Understanding user behavior is essential for the success of freemium businesses. By analysing how users interact with the free version of the product or service, companies can gain valuable insights into user preferences, habits, and pain points. This data enables businesses to tailor their premium offerings to address users’ needs effectively and maximise conversion rates.
Moreover, user behavior analysis allows freemium businesses to identify opportunities for upselling and cross-selling premium features to existing users. By tracking user interactions, engagement levels, and conversion pathways, companies can implement targeted marketing strategies to encourage free users to upgrade and enhance their overall experience.
In conclusion, the freemium business model presents an innovative approach to monetisation that relies on offering a free version of the product or service to attract users and drive conversions. By understanding the psychology behind user engagement and behaviour analysis, businesses can optimise their freemium strategies and unlock new revenue streams. Stay tuned for more insights on this fascinating topic!
Challenges in Analyzing Freemium User Behavior
Freemium business models have become increasingly popular in today’s digital landscape, offering users both free and paid services. However, analyzing freemium user behavior poses unique challenges due to the complexity of the data, the need to identify key behavioral metrics, and understanding the gap between free and paying users’ behavior.
Complexity in Freemium User Data
One of the primary challenges in analyzing freemium user behavior lies in the complexity of the data generated by these models. Freemium services typically cater to a large user base, including both free and paying users, each exhibiting varying levels of engagement and interaction with the platform.
- **Data Volume:** With a high volume of users accessing the free services, the amount of data generated can be overwhelming for analysis.
- **Data Variety:** Freemium models often involve multiple touchpoints, such as app usage, in-app purchases, and interactions with advertisements, leading to diverse data sources that need to be integrated and analyzed.
Understanding and making sense of this data requires advanced analytics tools and techniques to uncover valuable insights into user behavior and preferences.
Key Behavioral Metrics for Freemium Services
When analyzing freemium user behavior, it is essential to identify and track key behavioral metrics that provide valuable insights into user engagement, retention, and conversion. These metrics help businesses understand how users interact with the platform and make informed decisions to optimize the freemium model.
- **User Acquisition:** Tracking the number of new users signing up for the platform provides insights into the effectiveness of marketing strategies and user acquisition channels.
- **Engagement Metrics:** Monitoring user activity, such as session length, frequency of visits, and feature usage, helps measure user engagement and identify popular features driving retention.
- **Conversion Rate:** Analyzing the percentage of free users upgrading to paid subscriptions indicates the success of the freemium model in converting users to paying customers.
- **Churn Rate:** Calculating the rate at which users stop using the platform helps in understanding user dissatisfaction and improving retention strategies.
By focusing on these key behavioral metrics, businesses can gain insights into user behaviour patterns, identify trends, and tailor their strategies to enhance user experience and drive revenue growth.
Gap Between Free and Paying Users’ Behavior
One of the significant challenges in analyzing freemium user behavior is bridging the gap between the behavior of free users and paying customers. Free users often have different motivations, usage patterns, and expectations compared to paying customers, making it crucial to understand and address this disparity.
**Personalization:** Tailoring the user experience based on individual preferences and behaviour can help bridge the gap between free and paying users, improving engagement and conversion rates.
**Incentives:** Offering targeted incentives, such as exclusive features or discounts, can encourage free users to upgrade to premium services, closing the gap between the two user groups.
By analyzing the behavior of both free and paying users, businesses can gain a comprehensive understanding of their user base, identify opportunities for monetization, and optimize the freemium model to drive long-term success.
Successfully analyzing freemium user behavior requires a deep understanding of the data complexities, a focus on key behavioral metrics, and strategies to bridge the gap between free and paying users. By overcoming these challenges, businesses can effectively harness the power of freemium models to drive growth and maximize user value.
AI and Machine Learning in Behavioral Analytics
Introduction to AI and Machine Learning Concepts
Artificial Intelligence (AI) and Machine Learning have become buzzwords in the tech industry, but what do they really mean? In simple terms, AI refers to the simulation of human intelligence processes by machines, whereas Machine Learning is a subset of AI that allows machines to learn from data without being explicitly programmed. These technologies have revolutionized the way we analyze and understand user behavior.
Imagine having the ability to decipher intricate patterns in user actions and preferences, all thanks to the power of AI and Machine Learning. By harnessing these technologies, businesses can unlock valuable insights that can significantly enhance their decision-making processes.
AI Processing and Analyzing Large Datasets of User Behavior
One of the most significant advantages of AI in behavioral analytics is its prowess in processing and analyzing vast datasets of user behavior. Traditional methods often fall short when it comes to handling the sheer volume and complexity of data generated by users in today’s digital landscape.
AI algorithms excel at detecting correlations and patterns within these datasets that would be virtually impossible for humans to identify. By leveraging AI for data analysis, businesses can gain a comprehensive understanding of user behavior, leading to more targeted marketing strategies and personalized user experiences.
Furthermore, AI’s capability to process real-time data enables businesses to make instant adjustments to their strategies based on the most up-to-date insights. This agility is crucial in an era where user preferences and trends evolve rapidly.
Case Studies of AI Applications in Freemium User Analytics
Let’s delve into some real-world examples of how AI is revolutionizing freemium user analytics:
- Netflix: The streaming giant uses AI algorithms to analyze user behavior and recommend personalized content based on individual viewing habits. This not only enhances user satisfaction but also increases user retention rates.
- Spotify: By leveraging Machine Learning, Spotify provides users with tailored playlists and recommendations, creating a more engaging music-streaming experience. This personalized approach keeps users hooked on the platform.
- Google: Google’s search algorithms constantly evolve with the help of AI to deliver more relevant search results to users. By understanding user intent and behavior, Google enhances the overall search experience for millions of users worldwide.
These case studies underscore the transformative impact of AI in freemium user analytics, demonstrating how businesses can leverage these technologies to drive user engagement and loyalty.
AI-Driven Strategies for Enhancing User Engagement and Conversion
Welcome to this insightful discussion on AI-driven strategies for enhancing user engagement and conversion. In today’s digital age, leveraging artificial intelligence holds immense potential in transforming user interactions and driving conversions. Let’s delve into three key AI-driven techniques that can revolutionize user engagement and boost conversion rates.
1. Personalization and Recommendation Algorithms for User Engagement
Personalization has become a cornerstone of modern marketing strategies. By harnessing AI-powered recommendation algorithms, businesses can create tailored user experiences that resonate with individual preferences and behaviours.
Imagine visiting an e-commerce website and being greeted with product recommendations based on your previous purchases, browsing history, and demographic information. This level of personalization not only enhances user engagement but also increases the likelihood of conversion.
AI algorithms analyze vast amounts of data to predict user preferences accurately. By understanding customers’ interests and anticipated needs, businesses can deliver targeted content, offers, and product suggestions in real-time. This proactive approach not only increases user satisfaction but also cultivates brand loyalty.
2. Predictive Analytics for Identifying Potential Premium Subscribers
Predictive analytics empowers businesses to forecast future trends and outcomes by mining historical data and identifying patterns. When applied to user engagement and conversion, predictive analytics can help businesses pinpoint potential premium subscribers and tailor their marketing strategies accordingly.
For instance, a streaming service can use predictive analytics to identify users who are likely to upgrade to a premium subscription based on their viewing habits, engagement levels, and interactions with the platform. By targeting these high-value customers with personalized offers or exclusive content, the business can effectively drive conversions and maximize revenue.
Furthermore, predictive analytics enables businesses to segment their audience effectively, allowing for targeted marketing campaigns that resonate with specific customer segments. By leveraging insights derived from predictive models, businesses can optimize their conversion funnels and create compelling value propositions that attract premium subscribers.
3. AI-Based Churn Prevention Techniques
Churn, or the rate at which customers discontinue their engagement with a product or service, poses a significant challenge for businesses seeking to maintain a loyal customer base. AI-based churn prevention techniques leverage machine learning algorithms to identify at-risk customers and implement proactive strategies to retain their loyalty.
By analyzing user behaviour, engagement patterns, and feedback signals, AI algorithms can predict when a customer is likely to churn and intervene before it happens. This could involve sending personalized retention offers, providing proactive customer support, or enhancing the overall user experience to address potential pain points.
AI-based churn prevention techniques not only help businesses reduce customer attrition but also foster long-term customer relationships. By anticipating and mitigating churn risks, businesses can bolster user engagement, drive conversions, and ultimately, optimize their revenue streams.
In conclusion, AI-driven strategies offer a multitude of opportunities for enhancing user engagement and conversion. By embracing personalization, predictive analytics, and churn prevention techniques, businesses can create meaningful interactions, drive conversions, and cultivate lasting customer relationships in today’s competitive marketplace.
Ethical Considerations and Future Directions
Welcome back, readers! In this final section, we delve into the ethical considerations and future directions surrounding AI-powered analytics. Let’s discuss the importance of addressing privacy concerns, the significance of transparency in AI applications, and explore the exciting future trends in AI and user behavior analysis.
Addressing Privacy Concerns in AI-powered Analytics
Privacy concerns are a hot topic when it comes to AI-powered analytics. As these technologies become more prevalent in our daily lives, it’s crucial to address the potential risks to privacy. Organizations must prioritize the ethical collection, storage, and use of data to ensure user trust and compliance with data protection regulations.
By implementing robust data anonymization techniques, encryption protocols, and strict access controls, businesses can mitigate privacy risks associated with AI analytics. Transparency about data usage and providing users with clear consent mechanisms are essential steps in building a trustworthy relationship with customers.
Importance of Transparency in AI Applications
Transparency is key to fostering trust in AI applications. Users should have a clear understanding of how AI algorithms make decisions that affect them. By promoting transparency, organizations can improve accountability, mitigate bias, and promote fairness in AI systems.
Explainable AI (XAI) techniques aim to make complex AI algorithms more understandable to non-experts. By providing insights into the decision-making process, users can have more confidence in the outcomes generated by AI applications. Transparency also enables users to challenge and rectify any biased or unfair decisions made by AI systems.
Future Trends in AI and User Behavior Analysis
The future of AI and user behavior analysis is full of exciting possibilities. As advancements in AI technologies continue to evolve, we can expect more personalized experiences, improved predictive analytics, and enhanced user engagement. AI algorithms will become more adept at understanding user preferences, emotions, and behavior, leading to hyper-personalized recommendations and services.
Furthermore, the integration of AI with other emerging technologies such as Internet of Things (IoT) and augmented reality (AR) will revolutionize user behavior analysis. Real-time data insights and predictive modelling will enable organizations to anticipate user needs and deliver highly customized experiences.
TL;DR
In this final section, we explored the ethical considerations and future directions of AI-powered analytics. Addressing privacy concerns, ensuring transparency in AI applications, and embracing future trends in user behavior analysis are essential for fostering trust, accountability, and innovation in the AI landscape.
References
Prominent examples of businesses utilizing the freemium model include Slack, Dropbox, Spotify, Zoom, and Canva. Each of these companies offers basic services for free, with premium features available for a subscription fee. For instance, Slack allows users to access basic functionality indefinitely, while Dropbox offers a certain amount of storage for free, enticing users to upgrade for more space. Similarly, Spotify provides an ad-supported free tier with the option for a premium subscription for an ad-free experience, and Zoom offers free video conferencing with limitations that can be expanded upon subscribing to their paid plans. Canva, a graphic design platform, also operates on this model, offering free access with the option to upgrade for more advanced featureshttps://builtin.com/product/freemium
The freemium model can take various forms, including the traditional model where the basic service is free forever but with limited features, the “Land and Expand” model particularly effective for B2B companies, “Freeware 2.0” often seen in mobile games with optional paid upgrades, the “Ecosystem” model which involves third-party developers or apps paying, and the “Alternative Product Strategy” where a free product is used to introduce users to other paid offerings https://rockcontent.com/blog/examples-of-freemium-models/
There are both advantages and disadvantages to the freemium model. On the plus side, it helps attract a large user base, acts as a marketing tool through user recommendations, focuses on building long-term relationships with customers, and allows for multiple revenue streams. However, converting free users to paying customers can be challenging, and the model might lead to perceptions of lower quality. Additionally, maintaining the free version can be financially taxing if it does not lead to sufficient premium upgrades or other monetization methods https://www.saasgenius.com/blog-business/freemium-business-model-definition/
For more detailed insights and examples on the freemium model, you can explore the comprehensive discussions on these websites:
These sources provide an in-depth look at how companies have successfully navigated the challenges and opportunities presented by the freemium business model, from strategic implementation to overcoming common pitfalls.