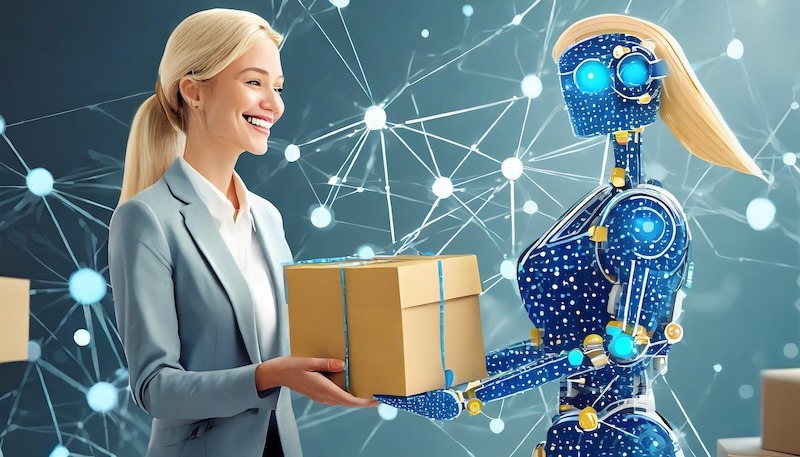
Understanding AI in the Context of Product Recommendations
Welcome to the world of Artificial Intelligence (AI), where machines are becoming smarter and more capable of making decisions that were once exclusively within the realm of human expertise. One area where AI has made significant advancements is in product recommendation systems. Today, AI is transforming the way businesses provide personalized suggestions to their customers, leading to improved customer satisfaction and increased sales.
Explanation of Artificial Intelligence and its Application in Product Recommendation Systems
Artificial Intelligence, in simple terms, refers to the ability of a computer or machine to mimic certain aspects of human intelligence. AI-driven recommendation systems leverage complex algorithms and machine learning techniques to analyze and understand vast amounts of customer data.
These recommendation systems use various types of AI models, such as collaborative filtering, content-based filtering, and hybrid models, to generate personalized product suggestions. Collaborative filtering analyzes the historical preferences of similar users to recommend items that a customer might like based on the preferences of others with similar tastes. Content-based filtering focuses on the attributes of the products themselves and recommends items based on their similarity to products the customer has already shown interest in. Hybrid models combine both approaches to provide even more accurate suggestions.
How AI Processes Customer Data to Generate Personalized Suggestions
The key to AI-driven recommendation systems lies in their ability to understand and process customer data. These systems collect and analyze various types of data, including past purchases, browsing history, wishlist items, demographics, and even contextual information like location and time of day.
AI algorithms then use this data to create customer profiles and develop insights about individual preferences and needs. These profiles are continuously updated and refined as customers interact with the platform. AI systems also consider factors like product popularity, inventory availability, and business goals to make the most relevant and contextually appropriate recommendations to each customer.
Case Studies or Examples of Successful AI-driven Recommendation Systems
Several companies have successfully implemented AI-driven recommendation systems, yielding impressive results. One notable example is Amazon, which uses AI algorithms to personalize product recommendations based on customer browsing and purchasing history. This approach has boosted their sales by a significant margin and improved customer satisfaction.
Netflix is another company that heavily relies on AI for their recommendation engine. By analyzing users’ viewing history and patterns, Netflix suggests personalized content, leading to increased engagement and customer loyalty.
Spotify utilizes AI to curate personalized music playlists for its users. Their recommendation system considers factors like listening history, preferred genres, and even the user’s mood to create bespoke playlists that enhance the user experience.
These case studies demonstrate the immense potential of AI-driven recommendation systems in enhancing customer engagement and driving business growth. By leveraging AI technology, businesses can provide seamless and personalized experiences to their customers, ultimately leading to increased customer satisfaction and long-term success.
The Impact of AI on Consumer Behavior and Preferences
Artificial Intelligence (AI) has become a transformative force in various industries, and its impact on consumer behavior and preferences cannot be overlooked. With advancements in AI technology, businesses can gain valuable insights into customer shopping behavior, understand and adapt to changing preferences, and provide personalized recommendations. In this blog post, we will explore the analysis of how AI influences customer shopping behavior, the role of AI in understanding and adapting to changing consumer preferences, and the discussion of consumer response to AI-driven recommendations.
Analysis of How AI Influences Customer Shopping Behavior
AI has revolutionized the way consumers make purchasing decisions. With the help of machine learning algorithms, AI systems can analyze vast amounts of data, including browsing history, purchase patterns, and social media interactions, to understand the preferences, needs, and desires of individual consumers. This analysis enables businesses to offer personalized product recommendations, customized marketing messages, and tailored shopping experiences.
Furthermore, AI-powered chatbots and virtual assistants provide real-time support and recommendations to customers, enhancing their shopping experience. This level of personalization enhances customer satisfaction, increases brand loyalty, and ultimately influences their shopping behavior.
The Role of AI in Understanding and Adapting to Changing Consumer Preferences
Consumer preferences are constantly evolving, and businesses need to keep up with these changes to remain competitive. AI plays a crucial role in understanding and adapting to these changing preferences. By analyzing consumer data and trends, AI systems can identify shifts in consumer preferences and anticipate future buying patterns.
AI algorithms can also predict consumer behavior based on past interactions and preferences. This enables businesses to align their product offerings, marketing strategies, and pricing models with consumer preferences, leading to increased customer satisfaction and loyalty.
Discussion of Consumer Response to AI-Driven Recommendations
Consumers have responded positively to AI-driven recommendations. Studies have shown that personalized product recommendations based on AI algorithms significantly influence purchase decisions. According to a survey conducted by Accenture, 66% of consumers are willing to switch brands to receive personalized recommendations.
AI recommendations not only save time and effort for consumers by offering relevant choices but also create a sense of trust and reliability. With the increasing sophistication of AI algorithms, these recommendations are becoming more accurate, leading to higher customer satisfaction and repeat purchases.
In conclusion, AI has a profound impact on consumer behavior and preferences. By analyzing customer shopping behavior, understanding and adapting to changing preferences, and providing AI-driven recommendations, businesses can enhance customer satisfaction, build brand loyalty, and ultimately drive revenue. As AI technology continues to advance, businesses must continue to leverage its capabilities to stay ahead in today’s competitive market.
Technological Foundations of AI-Driven Recommendations
AI-driven recommendation systems have become an integral part of our online experiences, providing personalized suggestions for products, movies, music, and more. These systems are powered by a variety of advanced technologies, including machine learning, natural language processing, and big data analytics. In this blog, we will take a detailed look at the technological foundations that make AI-driven recommendations possible, and how these technologies analyze customer data to provide accurate and relevant suggestions.
Machine Learning
Machine learning is at the core of AI-driven recommendation systems. It is a subset of artificial intelligence that allows computers to learn and make predictions or decisions without being explicitly programmed. In the context of recommendation systems, machine learning algorithms are trained on massive amounts of data, including customer preferences, purchase history, and browsing behavior. These algorithms analyze patterns and trends in the data and use this information to predict which items or content a user is likely to be interested in.
Natural Language Processing
Natural language processing (NLP) is another important technology used in recommendation systems. NLP focuses on understanding and interpreting human language, allowing computers to understand and respond to text or speech inputs. In the context of recommendations, NLP is used to analyze customer reviews, product descriptions, and other textual data to extract meaningful insights. By understanding the sentiment, context, and intent behind the text, recommendation systems can provide more accurate and relevant suggestions to users.
Customer Data Analysis
To analyze customer data, recommendation systems employ various techniques such as collaborative filtering, content-based filtering, and hybrid models. Collaborative filtering compares users’ preferences and behaviors to find similarities and recommend items that other users with similar tastes have liked. Content-based filtering, on the other hand, focuses on the features and attributes of items to suggest similar ones. Hybrid models combine both techniques to provide more accurate and diverse recommendations.
In addition to purchase history and browsing behavior, recommendation systems also consider contextual information such as location, time, and device type. By taking into account the user’s current context, these systems can offer recommendations that are more relevant and timely.
The Role of Big Data
Big data plays a significant role in enhancing the accuracy of AI recommendations. Recommendation systems analyze massive amounts of data from diverse sources, including user interactions, feedback, and external data such as social media trends. By processing and analyzing this vast volume of data, recommendation algorithms can uncover hidden patterns and correlations that might not be apparent with smaller datasets. This allows for more precise and personalized recommendations, improving the overall user experience.
In conclusion, AI-driven recommendation systems rely on a combination of advanced technologies such as machine learning, natural language processing, and big data analytics. These technologies enable recommendation systems to analyze customer data and provide accurate and relevant suggestions. By continuously learning and adapting to user preferences, AI-driven recommendation systems are constantly improving their ability to deliver personalized recommendations that enhance user satisfaction and engagement.
Benefits and Challenges of AI-Driven Product Recommendations
Artificial Intelligence (AI) has revolutionized various aspects of our lives, including the way businesses interact with consumers. One notable application of AI is in the realm of product recommendations. By analyzing vast amounts of consumer data, AI-driven recommendation systems can provide personalized suggestions that enhance user experience and ultimately drive sales.
Advantages of AI-Driven Product Recommendations
AI brings several benefits to both businesses and consumers:
- Enhanced Personalization: AI algorithms can analyze user behavior, preferences, and purchase history to provide highly tailored recommendations. This personalization not only improves user experience but also increases the chances of conversion and customer satisfaction.
- Increased Sales: By suggesting relevant products based on user preferences, AI-driven recommendation systems can effectively cross-sell and upsell, leading to increased sales and revenue for businesses.
- Improved Customer Retention: Personalized recommendations foster a sense of loyalty and engagement among consumers, increasing their likelihood of returning to the platform. This can significantly improve customer retention and lifetime value.
- Time and Cost Savings: AI automates the process of analyzing vast amounts of data, making it more efficient and cost-effective than manual methods. This enables businesses to make data-driven decisions faster, saving time and resources.
Challenges and Limitations in Implementing AI-Driven Recommendations
Despite the numerous benefits, AI-driven recommendation systems also face challenges and limitations:
- Data Quality: AI models heavily rely on high-quality and accurate data. Poor data quality, such as incomplete or biased datasets, can lead to inaccurate recommendations and potential customer dissatisfaction.
- Privacy Concerns: Collecting and leveraging customer data to provide personalized recommendations raises privacy concerns. Businesses must ensure transparent data handling practices and comply with relevant privacy regulations to build trust with consumers.
- Algorithmic Bias: AI algorithms may inadvertently perpetuate biases present in the data they are trained on. If left unchecked, this can result in discriminatory recommendations that negatively impact certain groups of users.
- User Acceptance: Some users may be skeptical or uncomfortable with AI-driven recommendations, perceiving them as intrusive or manipulative. Businesses need to communicate the value and transparency of their recommendation systems to gain user acceptance.
Ethical Considerations and Privacy Concerns
As AI-driven recommendation systems rely on customer data, ethical considerations and privacy concerns must be carefully addressed:
- Transparency: Businesses should be transparent about the data they collect, how it is used, and the benefits customers can expect from personalized recommendations.
- Consent: Obtaining informed consent from users for data collection and utilization is crucial. Users should have control over their data and the ability to opt out if desired.
- Data Security: Robust security measures must be implemented to safeguard customer data from unauthorized access or breaches.
- Regulatory Compliance: Businesses should comply with relevant data protection regulations, such as the General Data Protection Regulation (GDPR), to ensure the ethical and legal use of customer data.
In conclusion, AI-driven product recommendations offer significant advantages in terms of personalization, increased sales, customer retention, and cost savings. However, challenges related to data quality, privacy concerns, algorithmic bias, and user acceptance need to be addressed. By adopting transparent and ethical practices, businesses can harness the power of AI-driven recommendations while maintaining the trust and satisfaction of their customers.
Case Studies of AI in Action
In today’s rapidly evolving digital landscape, artificial intelligence (AI) has emerged as a powerful tool for businesses to enhance their decision-making processes and streamline operations. From personalized product recommendations to automated customer service, AI-driven recommendations have revolutionized the way companies engage with their customers. This blog section explores real-life case studies where AI-driven recommendations have been successfully implemented, showcasing the impact and potential of this technology.
In-depth Analysis of Specific Case Studies
One of the key aspects of this blog section is to provide an in-depth analysis of specific case studies where AI-driven recommendations have proven to be successful. Each case study will be examined, focusing on the challenges faced, the AI solution implemented, and the results achieved. By delving into the details, readers can gain a comprehensive understanding of how AI-driven recommendations can address unique business needs and deliver tangible benefits.
Through a critical examination of these case studies, patterns and commonalities can emerge. Such insights can help businesses identify the key factors that contribute to the success of AI-driven recommendations. By leveraging this knowledge, companies can avoid common pitfalls and optimize their strategies for implementing AI-driven recommendations effectively.
Lessons Learned and Best Practices
Building on the analysis of the case studies, this section will highlight the lessons learned and best practices identified from the successful implementation of AI-driven recommendations. These insights will offer practical guidance to organizations looking to harness the power of AI in their own operations.
Some key lessons that may arise include the importance of quality data, the need for continuous monitoring and optimization, and the significance of user feedback. By understanding these lessons and following best practices, businesses can increase the chances of successfully implementing AI-driven recommendations and maximizing their return on investment.
Future Prospects and Potential Advancements
The final part of this blog section will explore the future prospects and potential advancements in AI-driven recommendations based on the case studies examined. AI is a rapidly evolving field, and this section will highlight emerging trends and innovations that could shape the future of AI-driven recommendations.
Topics such as the integration of conversational AI, the use of reinforcement learning algorithms, and the ethical considerations surrounding AI recommendations will be explored. By looking ahead, businesses can gain insights into the potential of AI-driven recommendations and prepare for the future.
In conclusion, this blog section aims to provide readers with a deep understanding of AI-driven recommendations through real-life case studies. By analyzing specific examples, discussing lessons learned and best practices, and exploring future prospects, businesses can stay informed and make informed decisions about implementing AI-driven recommendations in their own operations.
Preparing for the Future of AI-Driven Recommendations
Artificial Intelligence (AI) has revolutionized the way businesses operate, especially when it comes to product recommendation systems. By leveraging sophisticated algorithms and machine learning, AI enables businesses to provide personalized recommendations to their customers, enhancing the overall customer experience. As technology continues to evolve, it is crucial for businesses to stay ahead of the curve and prepare for the future of AI-driven recommendations.
Strategies for businesses to integrate AI into their product recommendation systems
Integrating AI into product recommendation systems can significantly benefit businesses by increasing customer engagement, driving sales, and improving customer satisfaction. Here are some strategies businesses can implement:
- Data collection and analysis: Businesses must gather relevant data about their customers’ preferences, behaviors, and purchasing patterns. Analyzing this data will provide valuable insights that can be utilized to deliver personalized recommendations.
- Machine learning algorithms: Implementing machine learning algorithms is crucial for understanding complex customer preferences. By developing algorithms that can analyze vast amounts of data, businesses can create accurate and effective recommendation systems.
- Real-time updates: To ensure the relevancy of recommendations, businesses should use real-time data to update their recommendation systems. This allows for immediate adjustments based on changing customer preferences.
- A/B testing: Testing different recommendation algorithms and strategies can help businesses optimize their systems. By conducting A/B tests, businesses can identify the most effective approaches for improving their recommendation algorithms.
The role of ongoing research and development in AI for future enhancements
Ongoing research and development in AI will be instrumental in advancing product recommendation systems and personalization. As technology continues to evolve, businesses must invest in research and development to stay competitive. Here’s how ongoing research and development can enhance AI-driven recommendations:
- Improved algorithms: Researchers are constantly exploring new algorithms that can provide more accurate and personalized recommendations. Ongoing research will lead to the development of more sophisticated algorithms that can better understand customer preferences.
- Enhanced data analysis: Ongoing research in data analysis techniques will enable businesses to extract deeper insights from customer data. By utilizing advanced data analysis methods, businesses can uncover hidden patterns and trends that can further enhance their recommendation systems.
- Integration of new technologies: Research and development efforts focus on integrating emerging technologies, such as natural language processing and computer vision, into recommendation systems. This integration will enable businesses to deliver more contextual and personalized recommendations.
Predictions for the future of AI in product recommendation and personalization
As AI continues to evolve, the future of product recommendation and personalization looks promising. Here are some predictions for the future:
“Hyper-personalization: AI will enable businesses to provide hyper-personalized recommendations to individual customers, taking into account their unique preferences, context, and behavior.”
“Seamless cross-platform recommendations: AI will enable businesses to deliver consistent and seamless recommendations across multiple platforms, such as websites, mobile apps, and smart devices.”
“Integration of AI in physical stores: AI will be integrated into physical stores, enhancing the in-store shopping experience by providing personalized recommendations and real-time offers.”
In conclusion, integrating AI into product recommendation systems requires businesses to adopt strategies such as data collection and analysis, machine learning algorithms, real-time updates, and A/B testing. Ongoing research and development in AI will play a vital role in enhancing recommendation systems through improved algorithms, enhanced data analysis techniques, and the integration of new technologies. The future of AI in product recommendation and personalization looks promising, with hyper-personalization, seamless cross-platform recommendations, and the integration of AI in physical stores being some of the expected advancements.
Conclusion
After exploring and analyzing the role of AI-driven product recommendations in the retail and e-commerce sectors, it is clear that this technology has immense potential and is transforming the way businesses interact with their customers.
Summarizing the key points discussed in the article
Throughout this article, we have seen how AI-powered algorithms can analyze vast amounts of customer data to create personalized and accurate product recommendations. These recommendations not only enhance the customer experience but also drive sales and revenue for businesses.
Additionally, we have discussed how AI can revolutionize the retail and e-commerce sectors by creating seamless and immersive shopping experiences. With AI advancements such as chatbots, virtual reality, and augmented reality, customers can interact with products in innovative ways, leading to increased customer satisfaction and brand loyalty.
The future outlook of AI-driven product recommendations
The future of AI-driven product recommendations is highly promising. As the technology continues to evolve and improve, we can expect more sophisticated algorithms that can understand and predict customer preferences with even greater accuracy. This will result in more personalized and targeted recommendations, leading to higher conversion rates for businesses.
Furthermore, AI will play a significant role in shaping the future of the retail and e-commerce sectors. With advancements in machine learning and natural language processing, AI-powered chatbots will be able to provide real-time customer support, answer queries, and assist customers throughout their shopping journey. This level of personalized assistance will greatly enhance customer satisfaction and drive business growth.
Final thoughts on the role of AI in shaping customer experiences and business strategies
AI has emerged as a game-changer in the world of retail and e-commerce. By harnessing the power of AI-driven product recommendations, businesses can create highly personalized and customer-centric experiences. This, in turn, leads to increased customer engagement, higher conversion rates, and ultimately, business success.
However, it is important for businesses to strike the right balance between automation and the human touch. While AI can provide invaluable insights and recommendations, it is human creativity and empathy that can truly differentiate a brand and create memorable customer experiences. By leveraging the strengths of both AI and human expertise, businesses can deliver exceptional customer experiences and drive long-term success.