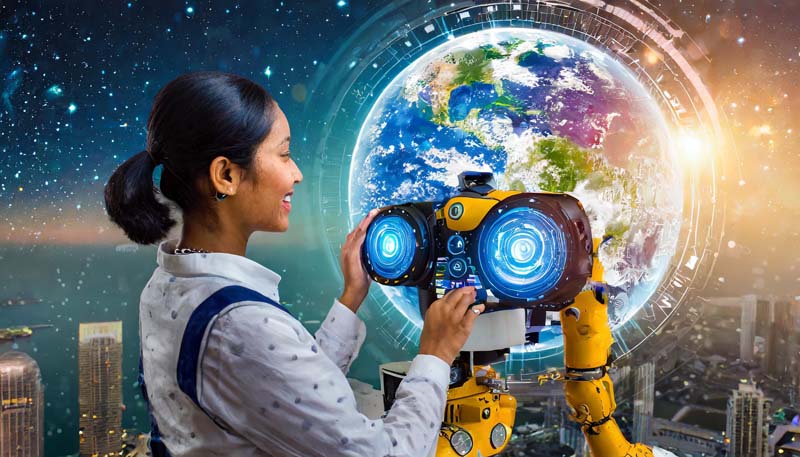
Understanding Marketing Data Quality
Welcome to the fascinating world of marketing data quality! In this section, we will delve into the intricacies of marketing data quality, exploring its definition, dimensions, impact on marketing strategies and outcomes, as well as common sources and quality issues faced by marketers.
Definition and Dimensions of Marketing Data Quality
Marketing data quality refers to the accuracy, completeness, consistency, reliability, and relevance of data used in marketing activities. It is crucial for marketers to ensure that the data they collect and analyse meets high-quality standards to make informed decisions and drive successful marketing campaigns.
There are several dimensions to consider when assessing marketing data quality:
- Accuracy: The data should be free from errors and reflect the true values of the variables being measured.
- Completeness: All relevant data points should be present without any missing values.
- Consistency: Data should be uniform and consistent throughout the dataset.
- Reliability: The data should be reliable and dependable for making marketing decisions.
- Relevance: Data should be relevant to the marketing objectives and goals.
Impact on Marketing Strategies and Outcomes
The quality of marketing data directly influences the effectiveness of marketing strategies and ultimately impacts the outcomes of marketing campaigns. Here’s how:
- High-quality data leads to more accurate customer insights, enabling marketers to target the right audience with personalised messages.
- Quality data enhances segmentation and targeting efforts, resulting in better engagement and conversion rates.
- Improved data quality ensures better campaign measurement and ROI tracking, enabling marketers to optimise their strategies in real-time.
- Poor data quality can lead to wasted resources, misguided decision-making, and failed marketing initiatives.
Common Sources and Quality Issues of Marketing Data
Marketers often encounter various sources of data and quality issues that can pose challenges to successful marketing campaigns:
- Internal Data: Data collected from sales reports, CRM systems, and customer interactions within the organization. Issues may arise from inconsistent data entry practices or outdated information.
- External Data: Information sourced from third-party vendors, market research reports, social media, and other external sources. Quality issues may stem from data inaccuracies or lack of validation.
- Data Integration: Combining data from multiple sources can lead to integration challenges, data discrepancies, and duplication errors.
- Data Governance: Lack of proper data governance policies and processes can result in data security breaches, compliance issues, and data silos.
By understanding the definition, dimensions, impact, and common issues of marketing data quality, marketers can take proactive steps to improve the quality of their data and drive successful marketing outcomes.“`
Key Criteria for Evaluating Data Quality
Welcome to the discussion on the key criteria for evaluating data quality. Data quality is vital for various aspects of decision-making, analysis, and operations within an organization. Evaluating data quality involves assessing several factors that impact the reliability and usefulness of data. In this blog section, we will explore the essential criteria for evaluating data quality and the tools and techniques available for measuring it.
Accuracy
One of the fundamental criteria for data quality is accuracy. Accurate data is free from errors and represents the real-world values or characteristics it intends to capture. Inaccurate data can lead to flawed analysis, incorrect conclusions, and poor decision-making. To ensure data accuracy, organisations should implement validation checks, data cleansing processes, and regular audits.
Completeness
Completeness refers to the extent to which all required data is present in a dataset. Incomplete data can hinder analysis and lead to biased results. To evaluate completeness, organisations should define the necessary data elements for a particular purpose and ensure that all relevant data is captured and maintained accurately.
Relevance
Relevance in data quality assessment pertains to the significance of the data in relation to the intended use or analysis. Irrelevant data can add noise and complexity to analyses, making it challenging to derive meaningful insights. Evaluating data relevance involves aligning data sources with the objectives of the analysis and ensuring that only pertinent data is included in the dataset.
Timeliness
Timeliness is a critical aspect of data quality, especially in fast-paced business environments. Timely data ensures that decisions are based on up-to-date information, enabling organizations to respond promptly to market changes and emerging trends. To assess data timeliness, organisations should establish data refresh schedules, monitor data sources for updates, and reduce processing delays.
Consistency
Consistency refers to the uniformity and standardisation of data across different sources, systems, and time periods. Inconsistent data can lead to discrepancies, duplication, and conflicting information, undermining the integrity of analyses. Evaluating data consistency involves comparing data from various sources, identifying discrepancies, and establishing data governance practices to maintain uniformity.
Tools and Techniques for Measuring Data Quality
Various tools and techniques are available to measure and assess data quality effectively. These include:
- Data Profiling: Data profiling tools analyse the structure, content, and quality of datasets to identify anomalies, patterns, and inconsistencies.
- Data Quality Metrics: Establishing data quality metrics allows organisations to quantify the level of data quality and track improvements over time.
- Data Cleansing Software: Data cleansing tools automate the process of identifying and correcting errors, duplicates, and inconsistencies in data.
- Statistical Analysis: Statistical techniques can help identify outliers, trends, and relationships in datasets, enabling the detection of data quality issues.
- Data Quality Dashboards: Interactive dashboards provide visual representations of data quality metrics, making it easier for stakeholders to monitor and assess data quality in real-time.
By leveraging these tools and techniques, organisations can enhance their data quality evaluation processes, improve decision-making, and drive better business outcomes.
Stay tuned for more insights on data quality management and best practices in our upcoming blog posts!
Steps to Evaluate Marketing Data Before Purchase
When it comes to enhancing your marketing strategies, one of the crucial steps is evaluating marketing data before making a purchase. The quality of your data can significantly impact the success of your campaigns. Here are some essential steps to ensure that you are investing in reliable and valuable marketing data:
Assess Data Quality Before Making a Purchase
Before you commit to purchasing any marketing data, it is imperative to assess the quality of the data. Poor-quality data can lead to ineffective targeting, wasted resources, and ultimately, unsuccessful marketing campaigns. Here are some factors to consider when evaluating data quality:
- Accuracy: Ensure that the data is accurate and up-to-date. Outdated or incorrect information can lead to misguided marketing decisions.
- Completeness: Check if the data provides comprehensive information that aligns with your marketing objectives. Incomplete data may hinder your ability to target the right audience effectively.
- Relevance: Consider whether the data is relevant to your target market and the specific goals of your marketing campaigns. Irrelevant data will not yield the desired results.
- Consistency: Verify the consistency of the data across different sources to ensure reliability and coherence in your marketing efforts.
By thoroughly assessing the quality of the data before making a purchase, you can avoid potential pitfalls and make informed decisions that benefit your marketing initiatives.
Conduct Preliminary Research on Data Vendors
Choosing the right data vendor is a critical step in the process of evaluating marketing data. Conducting preliminary research on potential data vendors can help you determine their credibility and the quality of the data they offer. Here are some tips for researching data vendors:
- Vendor Reputation: Look for reviews, testimonials, and case studies to gauge the reputation of the data vendor in the industry.
- Data Collection Methods: Inquire about the vendor’s data collection methods to ensure compliance with data privacy regulations and ethical standards.
- Data Sources: Ask about the sources of the vendor’s data to understand the reliability and relevance of the information provided.
- Data Updates: Inquire about the frequency of data updates to ensure that you are working with current and accurate data.
By conducting thorough research on data vendors, you can make an informed decision that aligns with your marketing goals and establishes a successful partnership.
Ask Vendors Questions to Ascertain Data Quality
Once you have narrowed down your options to a few potential data vendors, it is essential to ask them specific questions to assess the quality of their data. Here are some key questions to consider:
Can you provide examples of how your data has helped businesses achieve their marketing objectives?
How do you ensure the accuracy and relevance of your data?
What measures do you have in place to protect data privacy and comply with regulations?
Can you offer customizable data solutions tailored to our specific marketing needs?
Asking vendors these questions will enable you to gain insights into their data quality, capabilities, and compatibility with your marketing strategies. It is essential to establish clear communication and transparency with data vendors to build a trustworthy and beneficial partnership.
By following these steps to evaluate marketing data before making a purchase, you can enhance the effectiveness of your marketing campaigns, target the right audience, and achieve your desired outcomes successfully.
Maintaining Data Quality Post-Purchase
Welcome back! In this section, we will delve into the crucial strategies for maintaining data quality after acquisition. Ensuring that your data remains accurate, reliable, and valuable post-purchase is essential for the overall success of your business operations.
Regular Auditing and Cleaning of Data
One of the key strategies for maintaining data quality post-purchase is to conduct regular audits and cleaning processes. This involves reviewing the acquired data to identify any inconsistencies, errors, or outdated information that may have crept in over time.
By implementing a systematic approach to data auditing, you can ensure that your database remains up-to-date and free from inaccuracies. This may involve cross-referencing data against external sources, verifying the integrity of information, and rectifying any discrepancies that are discovered.
Automated data cleaning tools can also be utilised to streamline this process, helping to identify and rectify errors in a more efficient manner. By making data quality checks a routine part of your post-purchase operations, you can proactively address any issues that may compromise the integrity of your data.
Investing in Technology and Training for Data Quality Management
Another crucial aspect of maintaining data quality post-purchase is investing in the right technology and providing adequate training for your team members. Utilising advanced data management tools and software can help automate data quality processes, making it easier to monitor, cleanse, and enrich your datasets.
Training your employees on the best practices for data quality management is equally important. By ensuring that your team has the necessary skills and knowledge to handle data effectively, you can minimise the risk of errors and improve overall data accuracy.
Continuous education and upskilling in the field of data management can empower your employees to make informed decisions and maintain high standards of data quality. By investing in ongoing training and development, you can foster a culture of data excellence within your organisation.
Overall, maintaining data quality post-purchase requires a multi-faceted approach that combines regular auditing, data cleaning, technology investment, and employee training. By prioritising data quality management, you can enhance the value of your data assets and drive better business outcomes in the long run.
Challenges in Ensuring Data Quality
Welcome to our blog section on the challenges in ensuring data quality. In today’s data-driven world, maintaining high-quality data is crucial for business success and decision-making. Let’s dive into some common challenges in evaluating and maintaining data quality, explore the impact of evolving technology and big data, and discuss effective ways to address these challenges.
Common Challenges in Evaluating and Maintaining Data Quality
Ensuring data quality is an ongoing challenge for organisations of all sizes. Here are some common obstacles that businesses face when it comes to evaluating and maintaining data quality:
- Accuracy: One of the primary challenges is ensuring that the data collected is accurate and reliable. Inaccurate data can lead to incorrect insights and poor decision-making.
- Completeness: Another challenge is ensuring that the data is complete, with no missing values or gaps. Incomplete data can skew analysis results and hinder business operations.
- Consistency: Maintaining consistency across different datasets and data sources can be a challenge. Inconsistent data formats or definitions can lead to confusion and errors in analysis.
- Timeliness: Keeping data up to date in real-time is essential for businesses. Outdated data can impact the accuracy of reports and insights, leading to outdated decision-making.
- Relevancy: Ensuring that the data being collected is relevant to the business objectives is crucial. Irrelevant data can waste resources and cloud important insights.
Impact of Evolving Technology and Big Data
The rapid evolution of technology and the rise of big data have significantly impacted the challenges associated with ensuring data quality. As businesses collect and analyse vast amounts of data, new complexities arise, including:
- Data Volume: With the exponential growth of data volume, businesses struggle to manage and analyse huge datasets efficiently while maintaining data quality.
- Data Variety: Data now comes in various formats, including structured and unstructured data. Managing diverse data types poses a challenge in ensuring consistency and quality.
- Data Velocity: Real-time data processing and analysis have become essential for many businesses. Ensuring data quality in a fast-paced environment requires robust systems and processes.
- Data Complexity: Complex data relationships and dependencies can make it challenging to maintain data quality standards. Businesses need advanced tools and techniques to handle intricate data structures.
Effective Ways to Address Data Quality Challenges
While the challenges in ensuring data quality may seem daunting, there are effective strategies that businesses can implement to overcome these obstacles:
- Data Quality Assessment: Conduct regular audits and assessments to identify data quality issues and areas for improvement.
- Data Governance Framework: Implement a robust data governance framework to define data standards, policies, and procedures for maintaining data quality.
- Automated Data Quality Tools: Invest in automated data quality tools that can help in data profiling, cleansing, and monitoring to ensure high-quality data.
- Employee Training: Provide training to employees on data quality best practices and the importance of maintaining accurate and reliable data.
- Continuous Monitoring: Establish processes for continuous monitoring of data quality metrics and key performance indicators to detect and address issues promptly.
By proactively addressing data quality challenges and adopting best practices, businesses can enhance the reliability and integrity of their data, leading to more informed decision-making and improved business outcomes.
Conclusion
As we wrap up our discussion on data quality, it’s essential to reflect on the key points that highlight its importance in marketing strategies. By ensuring data accuracy, consistency, and relevance, businesses can make informed decisions that drive success. Let’s revisit the key takeaways, discuss the importance of prioritizing data quality evaluation, and envision the future of marketing data quality.
Recap of Data Quality Importance and Key Takeaways
Data quality is the foundation on which effective marketing strategies are built. It impacts every aspect of decision-making, from identifying target audiences to measuring campaign performance. Key takeaways include the need for clean and reliable data to drive actionable insights, improve customer relationships, and enhance overall business performance.
Encouragement to Prioritize Data Quality Evaluation
It’s crucial for businesses to prioritize data quality evaluation as an ongoing process rather than a one-time task. Regularly assessing data sources, cleansing data, and implementing quality control measures not only mitigates risks of errors but also boosts the efficiency and effectiveness of marketing efforts. By fostering a culture of data quality consciousness, organizations can stay ahead of the competition and drive sustainable growth.
Future Vision for Marketing Data Quality in Successful Strategies
Looking ahead, the future of marketing data quality lies in leveraging advanced technologies such as AI and machine learning to automate data validation processes and enhance data accuracy. Integrating data quality tools and practices into marketing automation platforms will streamline operations and empower marketers to make data-driven decisions with confidence. Embracing a proactive approach to data quality will be the cornerstone of successful marketing strategies in the digital age.
In conclusion, data quality is not just a technical requirement but a strategic asset that fuels business growth and drives marketing success. By recognising its significance, prioritising its evaluation, and embracing innovative solutions, businesses can unlock the full potential of their data assets and stay ahead in today’s competitive landscape.
References
Understanding and maintaining marketing data quality is essential for effective email marketing campaigns and overall marketing strategy success. Data quality affects various aspects of marketing, from customer relationships to decision-making and campaign personalization. High-quality data enables targeted messaging, accurate campaign tracking, and informed strategic decisions, leading to improved ROI and customer engagement.
Impact of Data Quality on Marketing Strategies
Data quality directly influences the effectiveness of marketing strategies. High-quality data leads to accurate customer insights, enabling personalized messaging and targeting, which in turn enhances customer engagement and conversion rates. Conversely, poor data quality can result in wasted resources and unsuccessful marketing efforts due to inaccurate targeting and decision-making. https://www.marketingevolution.com/marketing-essentials/data-quality https://seeresponse.com/blog/email-marketing-roi-data-list-quality/
Common Data Quality Issues in Email Marketing
Common data quality issues include outdated information, duplication, and incomplete data. These issues can lead to decreased ROI, as inaccurate or incomplete data may lead to messages being sent to non-existent recipients or failing to resonate with the intended audience. Ensuring data accuracy, completeness, and up-to-dateness is crucial for the success of email marketing campaigns. https://seeresponse.com/blog/email-marketing-roi-data-list-quality/
Best Practices for Maintaining Data Quality
- Regular Data Audits and Cleansing: Regular checks and audits are essential for maintaining high-quality data. This involves identifying and correcting inaccuracies, redundancies, and outdated information, which can skew campaign results and lead to erroneous decision-making.
- Clear Data Management Roles: Establishing clear responsibilities, such as Data Manager or Data Governance Manager roles, ensures that data collection and cleansing policies are created and adhered to, maintaining data quality across the organization https://www.marketingevolution.com/marketing-essentials/data-quality
Personalization and Accurate Targeting: High-quality data allows for effective personalization and segmentation of email campaigns, leading to higher engagement rates. Personalization can include using the recipient’s name or tailoring content to match their interests, significantly improving campaign success rates https://seeresponse.com/blog/email-marketing-roi-data-list-quality/
To overcome challenges associated with data quality, organizations should implement clear data governance policies, utilize technology for combining and analyzing diverse datasets, and employ data scientists for interpreting results. Establishing a clear process for data collection and formatting is also crucial for maintaining clean and accessible records. https://www.marketingevolution.com/marketing-essentials/data-quality